How AI is improving credit scoring systems
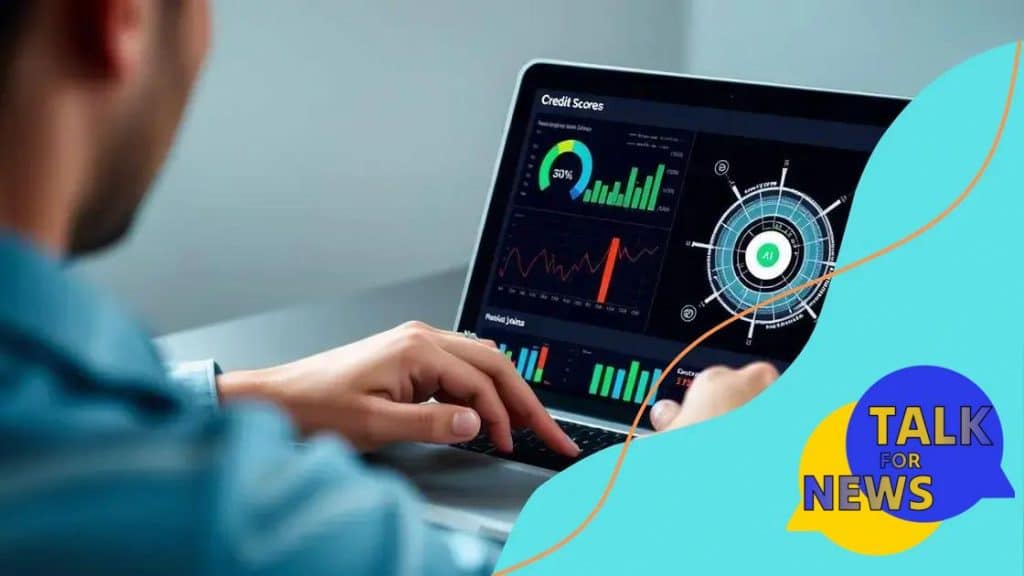
Anúncios
AI is improving credit scoring systems by enhancing accuracy and fairness through advanced data analysis, incorporating alternative data, and providing real-time insights for better lending decisions.
How AI is improving credit scoring systems is a game-changer for lenders and borrowers. Imagine a world where credit assessments are fairer and more accurate. Curious to see how this technology works? Let’s dive in!
Anúncios
Understanding traditional credit scoring systems
Understanding traditional credit scoring systems is essential for evaluating how they’re evolving with AI. These systems often rely on historical data to determine an individual’s creditworthiness. They use factors like payment history, credit utilization, and length of credit history. However, traditional methods can inadvertently disadvantage certain groups due to data limitations and biases.
Key Components of Credit Scoring
At the core of these systems, there are several key components that impact your credit score:
- Payment history: Timely payments boost your score, while missed payments can significantly lower it.
- Credit utilization: Using a smaller portion of available credit often results in a better score.
- Length of credit history: A longer credit history can positively influence your score, as it demonstrates reliability.
Anúncios
While these components are vital, they don’t tell the whole story. Many individuals with minimal credit history struggle to secure loans, even if they have the means to repay. This is where traditional systems can fall short, as they may not adequately reflect an individual’s financial behavior.
Limitations of Traditional Scoring
Moreover, traditional credit scoring can perpetuate existing inequalities. For instance, those from marginalized communities may lack access to credit entirely, leading to lower scores. This creates a cycle that is hard to break. Utilizing alternative data sources can enhance fairness in credit assessments.
Moreover, credit scoring must adapt to our changing economy. By incorporating innovations like machine learning, lenders can gain better insights into a borrower’s true creditworthiness, moving away from outdated methods. Adopting new standards and practices will enable financial institutions to make more informed and equitable lending decisions.
As we delve further into how AI is enhancing these systems, it becomes clear that the current models need a transformation. This is not just about accuracy in numbers; it’s also about ensuring justice in the financial landscape.
How AI enhances data analysis
AI technology is reshaping how we approach data analysis today. By using advanced algorithms, AI can process large sets of data quickly and effectively. This capability transforms raw data into useful insights.
Benefits of AI in Data Analysis
Utilizing AI for data analysis comes with several advantages:
- Speed: AI can analyze vast amounts of data in a fraction of the time it would take a human.
- Accuracy: With machine learning, AI reduces errors and improves the quality of insights derived from information.
- Predictive analytics: AI can forecast trends based on historical data, helping companies make informed decisions.
In addition to these benefits, AI empowers companies to identify various patterns within their data. For example, businesses can detect customer behaviors and preferences that were previously hidden. This information enhances marketing strategies and product development.
Real-Time Analysis
Another crucial aspect of AI is its ability to provide real-time data analysis. Businesses no longer have to wait for reports to understand their performance. They can receive instant feedback and adjust their strategies on the go. This immediate access to data allows for quick reactions to market changes.
Furthermore, AI’s ability to handle unstructured data is impressive. Images, text, and social media posts, which once posed challenges, can now be analyzed for insights integrating this information to enhance customer understanding and engagement.
As we look at how AI is transforming data analysis, it becomes evident that the future of decision-making lies in these intelligent systems. Companies that adopt AI tools will stay competitive by leveraging timely and relevant insights.
The role of machine learning in credit assessments
The role of machine learning in credit assessments is becoming increasingly important. This technology helps lenders analyze vast amounts of data to determine the creditworthiness of individuals and businesses. By learning from previous data, machine learning can improve accuracy and fairness in lending.
How Machine Learning Works
Machine learning uses algorithms to identify patterns in data. When applied to credit assessments, these algorithms can understand complex relationships between various factors. Here are some key aspects of how it improves the process:
- Data processing: Machine learning can process large datasets quickly, giving lenders insights faster.
- Pattern recognition: It identifies unusual patterns that may indicate risk or potential for default.
- Dynamic learning: As new data becomes available, machine learning systems can adapt and refine their models accordingly.
This ability to adapt leads to better decision-making. Traditional methods may ignore certain data points, while machine learning incorporates many factors, providing a holistic view of an applicant’s credit profile.
Reducing Bias in Lending
Another significant benefit of machine learning is its potential to reduce bias in lending decisions. By analyzing diverse data sources, it can create fairer assessments of applicants. This is especially vital for individuals from underrepresented backgrounds who may have been overlooked in traditional credit scoring systems.
The practical implications are vast. Lenders utilizing machine learning can make more informed decisions, reducing the chances of discrimination and promoting financial inclusion. Moreover, these systems can flag discrepancies or anomalies that might suggest unfair practices.
As we explore more about the integration of machine learning in credit assessments, it becomes essential to consider its ongoing evolution. With the right implementation, this technology creates a promising future for equitable lending.
Benefits of improved accuracy in scoring
Improved accuracy in scoring has significant benefits for both lenders and borrowers. When credit scoring systems become more accurate, businesses can make better lending decisions that take into account a wider range of data. This leads to a number of advantages.
Enhanced Risk Assessment
One major benefit is enhanced risk assessment. With accurate scores, lenders can identify low-risk borrowers more effectively. This means they can offer lower interest rates to those who are more likely to repay their loans. Additionally, it minimizes the risk of default, making the lending process safer.
- Better interest rates: Borrowers with higher scores receive better financing terms.
- Broader access to credit: More accurate assessments allow individuals with limited credit history to gain access.
- Lower default rates: More effective risk assessment leads to fewer defaults.
With accurate scoring, lenders can also uncover hidden opportunities. They can identify individuals who may not have received loans before but who now qualify based on their improved creditworthiness. This encourages inclusion and creates opportunities for economic growth.
Improved Financial Planning
Additionally, improved accuracy aids in financial planning for both consumers and lenders. Borrowers can better understand their credit situation, enabling them to make informed financial decisions. Lenders benefit from having accurate data for forecasting and marketing, leading to more effective strategies.
Furthermore, as lenders trust the accuracy of scores, they can streamline their processes, reducing time spent on manual checks. This efficiency ultimately results in a more positive experience for customers, enhancing trust and satisfaction.
As the accuracy of credit scoring continues to improve, we can expect significant changes in the lending landscape. Both lenders and borrowers reap the rewards of a more precise and fair system.
Future trends in AI and credit scoring
The future trends in AI and credit scoring promise to revolutionize the financial landscape. As technology advances, credit scoring systems will become more sophisticated, incorporating more data sources and improving decision-making processes.
Integration of Alternative Data
One significant trend is the integration of alternative data into credit scoring models. This data can include social media activity, utility payments, and even shopping habits. By using these additional sources, lenders can develop a more comprehensive view of a borrower’s financial behavior.
- Diverse data sources: Leveraging various data points improves accuracy.
- Access for underbanked populations: Alternative data can help those with limited credit histories qualify for loans.
- Personalized lending: Tailored offers based on unique borrower profiles enhance the lending experience.
Moreover, AI algorithms will evolve to analyze this diverse data more efficiently. As machine learning becomes more advanced, it will enable lenders to refine their models continuously and adapt to changing economic conditions.
Increased Use of Real-Time Data
Another trend involves the increased use of real-time data in credit assessments. As data flow in continuously, organizations can respond quickly to changes in a borrower’s financial situation. This dynamic approach can significantly enhance risk assessment.
In addition, lenders will adopt AI-driven strategies to customize products for consumers. By analyzing individual behavior, credit scoring models can recommend suitable financial products, creating a more user-centric experience.
Furthermore, the emphasis on transparency and ethical AI will grow. As consumers become more aware of how credit scoring affects their lives, transparency in the algorithms used and the data considered will be crucial. Ensuring fairness will not only build trust but also encourage broader participation in the financial system.
As we consider these trends, it’s clear that the future of AI in credit scoring is focused on inclusivity, fairness, and personalization. These advancements hold the potential to create a more equitable lending environment for all.
In conclusion, the integration of AI into credit scoring systems is transforming the financial landscape. Improved accuracy leads to better risk assessments, benefiting both lenders and borrowers. With trends like the inclusion of alternative data and real-time analytics, future credit assessments will be more precise and fair. Emphasizing transparency and ethical practices will create a more equitable environment, allowing everyone better access to credit. As we move forward, these advancements will not only enhance financial opportunities but also contribute to a healthier economy for all.
FAQ – Frequently Asked Questions about AI in Credit Scoring
How does AI improve the accuracy of credit scoring?
AI analyzes larger and more diverse data sets, leading to a more comprehensive understanding of a borrower’s creditworthiness.
What is the role of alternative data in credit assessments?
Alternative data helps provide a more complete picture of a borrower’s financial behavior, making credit access easier for those with limited history.
Why is real-time data important in credit scoring?
Real-time data allows lenders to make quicker, more informed decisions based on the most current financial information.
How can increased transparency in AI algorithms benefit borrowers?
Transparency builds trust and ensures that borrowers understand how their scores are calculated, reducing the risk of discrimination.