The role of AI in predicting stock market fluctuations
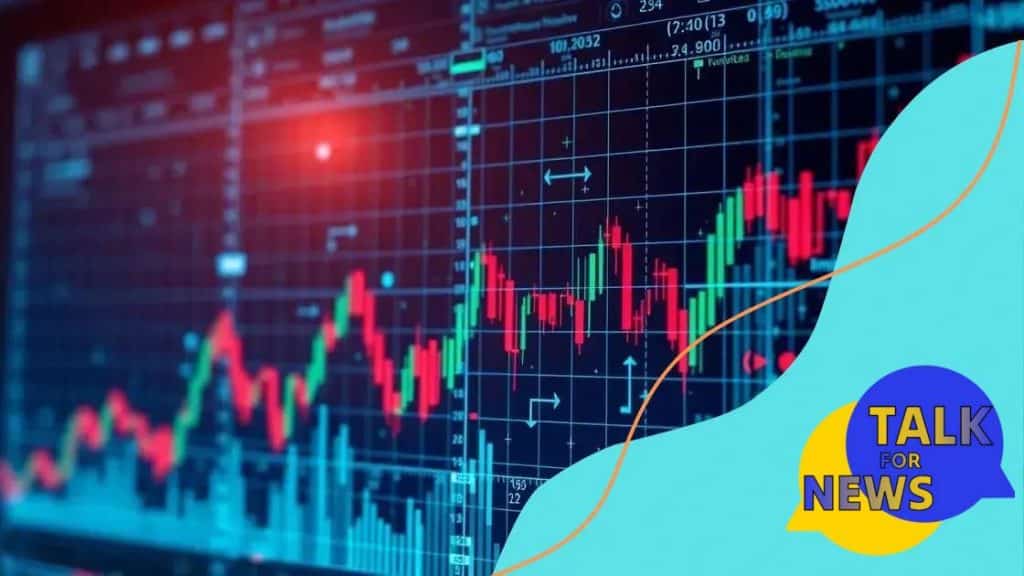
Anúncios
The role of AI in predicting stock market fluctuations significantly enhances trading strategies by analyzing vast amounts of data to identify patterns and make informed predictions.
The role of AI in predicting stock market fluctuations is reshaping how investors approach the market. Have you noticed how advanced algorithms now assist traders in making informed decisions? Let’s dive into this fascinating intersection of technology and finance.
Anúncios
Understanding stock market fluctuations
Understanding stock market fluctuations is essential for anyone interested in investing. These fluctuations are influenced by various factors that can create ups and downs in stock prices. Investors need to stay informed and prepared to navigate these changes.
Key Factors Influencing Stock Market Fluctuations
Several elements can impact the stock market, and recognizing these can help investors make informed decisions. The following are some critical factors:
- Economic Indicators: Data such as unemployment rates and GDP growth can significantly affect market sentiment.
- Interest Rates: The decisions made by central banks regarding interest rates often lead to market volatility.
- Market Sentiment: The emotional reactions of investors to news and events can cause sudden price changes.
- Global Events: Political instability, natural disasters, or major global events can create unexpected fluctuations.
Anúncios
Investors must also consider how companies perform financially. Earnings reports, new product launches, and shifts in management can lead to price changes. Monitoring these aspects allows traders to anticipate potential fluctuations.
The Impact of Technology on Understanding Trends
With advancements in artificial intelligence, analyzing stock trends has become more effective. Algorithms can process vast amounts of data quickly, providing insights that human analysts might overlook. They can identify patterns and trends based on historical data, offering valuable predictions.
The use of AI tools enhances the investor’s ability to interpret stock market movements. Machine learning can refine predictions, adjusting for market anomalies and creating more robust forecasting models. This technology is revolutionizing how traders approach stock market fluctuations.
In conclusion, recognizing and understanding stock market fluctuations requires a blend of knowledge about economic indicators and the use of technology. Staying informed enables investors to make smart choices, ultimately improving their chances of success in the market.
How AI analyzes historical market data
How AI analyzes historical market data is fascinating and transformative for investors today. When looking at the stock market, patterns can emerge from past data, helping traders make informed decisions. By using advanced algorithms, AI uncovers insights that traditional methods might miss.
The Role of Data in Analysis
Data is at the heart of AI analysis. Historical market data, including prices, trading volume, and economic indicators, provides the raw material for AI to work with. This data can reveal trends and correlations over time. Recognizing these patterns can help predict future movements in stock prices.
- Data Quality: High-quality and accurate historical data is crucial for reliable analysis.
- Volume of Data: The more data available, the better the AI can learn and identify trends.
- Types of Analysis: AI can perform both descriptive and predictive analysis, offering a comprehensive view.
AI employs various techniques to analyze this data effectively. Machine learning models can be trained on historical data, allowing them to improve and adapt over time. They can analyze multiple factors simultaneously, providing a deep understanding of complex relationships in the stock market.
Machine Learning Techniques
Different machine learning techniques are frequently utilized in market analysis. These include regression analysis, decision trees, and neural networks. Each method has its strengths in handling different types of data and can yield valuable insights.
For example, regression analysis can highlight relationships between different variables. In contrast, neural networks can identify nonlinear relationships and complex patterns. AI systems can quickly adapt and refine their approaches based on new data, ensuring up-to-date analysis.
Moreover, the speed at which AI can process data is unmatched. This capability allows traders to receive near real-time updates on market trends. The faster they can analyze and react to changes, the better their chances of success.
The role of machine learning in predictions
The role of machine learning in predictions is revolutionizing the way we understand market behavior. By employing algorithms, machine learning analyzes vast amounts of data. This process helps traders predict stock movements with greater accuracy.
How Machine Learning Works
Machine learning uses data to train models that can identify patterns. These models improve over time as they receive more data. For stock predictions, algorithms review historical prices, trading volumes, and various market factors. This allows them to make reliable forecasts regarding future prices.
- Supervised Learning: In this approach, models learn from labeled data, making predictions based on past outcomes.
- Unsupervised Learning: This method helps find hidden patterns in data without predefined labels, aiding in anomaly detection.
- Reinforcement Learning: Here, models learn through trial and error, optimizing their strategies based on feedback from past actions.
Machine learning also enhances the speed and efficiency of predictions. Unlike traditional methods that require manual analysis, machine learning processes data instantly. This real-time analysis enables traders to act quickly on potential opportunities.
Benefits of Machine Learning in Predictions
There are numerous benefits to using machine learning for stock predictions. One significant advantage is its ability to analyze large data sets that humans can’t process effectively. By identifying trends and shifts in market behavior, machine learning can help traders make informed decisions.
Furthermore, machine learning models can adjust to new data and changing conditions. As markets evolve, these models learn and adapt, maintaining their predictive accuracy. This adaptability is crucial for staying ahead in today’s volatile markets.
Overall, the integration of machine learning into stock predictions contributes to more effective trading strategies. As technology advances, the insights gained from these tools will continue to shape successful investing practices.
Successful case studies of AI in finance
Successful case studies of AI in finance demonstrate the remarkable impact this technology has on the industry. From banks to investment firms, AI is revolutionizing how financial institutions operate and make decisions. Companies implementing AI solutions have seen significant improvements in efficiency and accuracy.
Case Study: JPMorgan Chase
JPMorgan Chase utilizes AI for various applications, including fraud detection and customer service. Their AI-powered systems analyze vast data to detect irregularities in transactions. This proactive approach not only enhances security but also improves customer trust.
Case Study: BlackRock
BlackRock, one of the largest investment management firms, leverages machine learning algorithms to optimize its investment strategies. By analyzing historical market data, BlackRock’s AI identifies patterns that inform their investment decisions, helping them outperform competitors.
- Efficient Portfolio Management: AI tools enable better allocation of assets.
- Risk Assessment: Machine learning algorithms model different scenarios to assess risks more accurately.
- Personalized Client Solutions: AI helps tailor financial advice to individual client needs, enhancing satisfaction.
Another example includes Goldman Sachs, which employs AI for high-frequency trading. Their algorithms rapidly analyze market conditions and execute trades within milliseconds, capitalizing on fleeting opportunities. This speed and accuracy can significantly impact their overall profitability.
Impact on Lending
AI is also transforming the lending process. Companies like Zest AI use machine learning to assess credit risk more accurately. By evaluating a broader set of data points, including social media activity and payment histories, AI provides a more holistic view of a borrower’s creditworthiness.
This innovation not only reduces the default rates but also expands access to credit for individuals who may not have qualified under traditional scoring methods. As more financial institutions adopt these AI-driven solutions, the landscape of finance will continue to evolve.
Challenges and limitations of AI in stock trading
Challenges and limitations of AI in stock trading are critical to understand, even as this technology becomes more prevalent. While AI offers powerful tools for analysis and prediction, it is not without its issues. Investors need to be aware of these challenges to make informed decisions.
Data Quality and Availability
One primary challenge of AI in stock trading is the quality of data. AI algorithms depend heavily on large datasets to function effectively. If the data used for training is inaccurate or biased, the algorithms may produce unreliable predictions.
- Inconsistent Data: Inconsistent historical data can lead to flawed models.
- Limited Data Sources: Access to only a few data sources may restrict the AI’s ability to learn effectively.
- Noise in Data: Market noise can obscure signals, making it harder for AI to find actionable insights.
Additionally, AI models often require constant updates with fresh data. Rapid market changes mean that models can quickly become outdated. Therefore, regular maintenance and retraining are essential.
Market Dynamics and Human Factors
Another limitation is that AI may struggle to account for human behavior and emotional factors that drive market dynamics. While it excels at processing numbers, AI does not fully understand the narratives or sentiments behind market movements.
For instance, unexpected news events can lead to rapid price changes that AI models may not predict accurately. Moreover, high volatility periods can significantly challenge algorithms, as they often rely on historical data trends that may not hold true in such times.
Lastly, over-reliance on AI tools can lead investors to neglect fundamental analysis. A balanced approach that includes both AI and traditional investment strategies can provide better insights.
In conclusion, AI plays a significant role in stock market predictions, offering both opportunities and challenges. While the technology improves analysis and trading strategies, it cannot replace human intuition and understanding. Investors should balance AI tools with traditional methods. By being aware of data quality and market dynamics, traders can navigate the complex landscape of finance effectively. Embracing AI in the right way can lead to smarter investment decisions and greater success in the stock market.
FAQ – Frequently Asked Questions About AI in Stock Trading
How does AI improve stock market predictions?
AI analyzes vast amounts of historical data quickly, identifying patterns that can help predict future market movements.
What are the main challenges of using AI in stock trading?
The main challenges include data quality issues, market dynamics that AI may misinterpret, and the need for ongoing model updates.
Can AI fully replace human traders?
While AI can enhance trading strategies, human insights and intuition remain crucial for successful investing.
What role does data quality play in AI effectiveness?
High-quality data is essential; if the data is biased or inconsistent, the AI’s predictions can be unreliable.